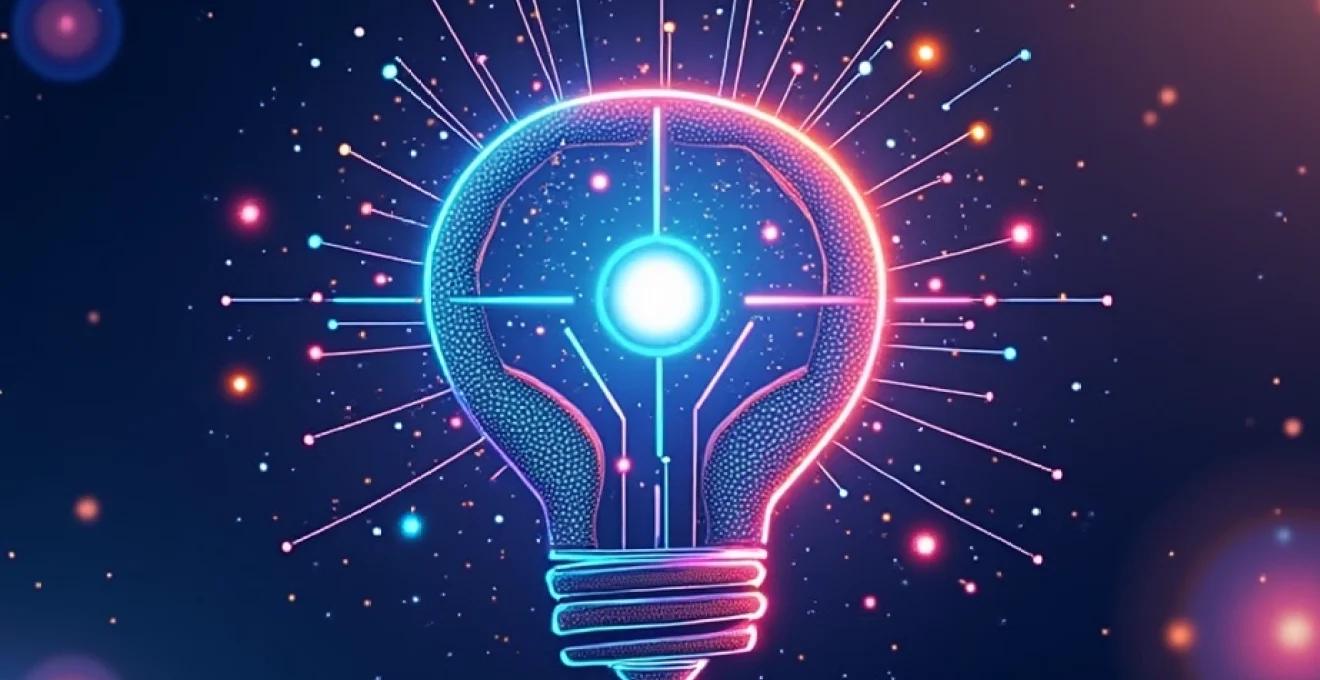
Artificial intelligence (AI) stands at the forefront of technological advancement, poised to revolutionize innovation across industries. As businesses and researchers push the boundaries of what's possible, AI emerges as a critical tool for solving complex problems and driving progress. From enhancing decision-making processes to automating intricate tasks, AI's potential to transform our world is immense. The convergence of machine learning, natural language processing, and computer vision is opening new frontiers in scientific discovery, product development, and service delivery.
The impact of AI on innovation is not just theoretical; it's already reshaping how we approach challenges in fields ranging from healthcare to finance, manufacturing to creative arts. By augmenting human capabilities and uncovering patterns in vast datasets, AI is accelerating the pace of innovation and enabling breakthroughs that were once thought impossible. As we delve into the key aspects of AI that are fueling this innovation revolution, it becomes clear that embracing and understanding these technologies is crucial for anyone looking to stay competitive in the rapidly evolving landscape of the 21st century.
Machine learning algorithms driving AI-powered innovation
At the heart of AI's innovative power lies machine learning algorithms. These sophisticated mathematical models enable computers to learn from data, improving their performance on specific tasks without being explicitly programmed. The evolution of machine learning has been nothing short of remarkable, with algorithms now capable of tackling increasingly complex problems across diverse domains.
Deep learning networks and neural architecture search
Deep learning, a subset of machine learning inspired by the human brain's neural networks, has emerged as a game-changer in AI innovation. These multilayered neural networks can automatically learn hierarchical representations of data, enabling them to perform tasks such as image recognition, speech processing, and natural language understanding with unprecedented accuracy.
Neural Architecture Search (NAS) represents the cutting edge of deep learning innovation. This meta-learning approach automates the design of neural network architectures, optimizing them for specific tasks. By leveraging NAS, researchers can discover novel network structures that outperform human-designed architectures, pushing the boundaries of AI capabilities even further.
Reinforcement learning for complex decision-making systems
Reinforcement learning (RL) has emerged as a powerful paradigm for training AI agents to make sequential decisions in complex environments. By learning through trial and error, RL algorithms can master tasks ranging from game-playing to robotic control. The success of RL in domains like strategic planning and resource allocation has opened new avenues for innovation in fields such as logistics, energy management, and autonomous systems.
One of the most exciting applications of RL is in the development of AI systems that can adapt to changing conditions and optimize their performance over time. This adaptability is crucial for creating intelligent systems that can operate effectively in real-world scenarios where conditions are often unpredictable and dynamic.
Generative adversarial networks (GANs) in creative AI applications
Generative Adversarial Networks represent a revolutionary approach to AI-driven content creation. GANs consist of two neural networks—a generator and a discriminator—that compete against each other, resulting in the creation of highly realistic synthetic data. This innovative technique has found applications in diverse fields, from art and design to data augmentation for machine learning models.
The impact of GANs on creative industries is profound. They enable the generation of photorealistic images, lifelike 3D models, and even coherent text, pushing the boundaries of what's possible in digital content creation. As GANs continue to evolve, they're opening up new possibilities for personalized content, virtual reality experiences, and automated design processes.
Natural language processing revolutionizing human-computer interaction
Natural Language Processing (NLP) is at the forefront of AI innovation, transforming how humans interact with machines. By enabling computers to understand, interpret, and generate human language, NLP is breaking down barriers between people and technology, paving the way for more intuitive and efficient communication interfaces.
Transformer models and BERT's impact on language understanding
The introduction of Transformer models, particularly BERT (Bidirectional Encoder Representations from Transformers), has marked a significant milestone in NLP. These models have revolutionized language understanding by capturing context and nuances in text with unprecedented accuracy. BERT's ability to process words in relation to all other words in a sentence, rather than one-by-one in order, has led to dramatic improvements in tasks such as question answering, sentiment analysis, and language translation.
The impact of BERT extends beyond academic research, finding applications in search engines, virtual assistants, and content recommendation systems. By improving machines' ability to understand human queries and content, BERT is enhancing user experiences across a wide range of digital platforms and services.
GPT-3 and large language models in content generation
GPT-3 (Generative Pre-trained Transformer 3) represents a quantum leap in natural language generation capabilities. As one of the largest and most powerful language models to date, GPT-3 can generate human-like text, answer questions, and even write code with remarkable coherence and relevance. Its ability to perform a wide variety of language tasks without fine-tuning has opened up new possibilities for automated content creation, personalized communication, and AI-assisted writing.
The emergence of GPT-3 and similar large language models is spurring innovation in fields such as journalism, customer service, and education. These models are enabling the creation of more sophisticated chatbots, automated report generation, and personalized learning experiences, demonstrating the transformative potential of AI in content-driven industries.
Sentiment analysis and emotion AI in customer experience
Sentiment analysis and emotion AI represent cutting-edge applications of NLP that are revolutionizing customer experience management. By analyzing text, speech, and facial expressions, these technologies can discern the emotional state and attitudes of customers, providing valuable insights for businesses to improve their products and services.
The integration of sentiment analysis into customer feedback systems, social media monitoring, and market research is enabling companies to respond more effectively to customer needs and preferences. This emotional intelligence layer added to AI systems is fostering more empathetic and personalized interactions between businesses and their customers, driving innovation in customer relationship management and service delivery.
Computer vision and image recognition enhancing perception systems
Computer vision, powered by advanced AI algorithms, is transforming how machines perceive and interact with the visual world. This technology is driving innovation across various sectors, from healthcare and automotive to retail and security, by enabling machines to interpret and analyze visual information with human-like accuracy.
Convolutional neural networks in object detection and classification
Convolutional Neural Networks (CNNs) have emerged as the cornerstone of modern computer vision systems. These specialized neural networks excel at processing grid-like data, making them ideal for tasks such as image classification, object detection, and semantic segmentation. The ability of CNNs to automatically learn hierarchical features from images has led to breakthroughs in fields ranging from medical imaging to autonomous driving.
In healthcare, CNNs are revolutionizing diagnostic processes by analyzing medical images to detect diseases with accuracy rivaling that of human experts. In manufacturing, these networks are powering quality control systems that can identify defects at speeds and scales impossible for human inspectors. The versatility and effectiveness of CNNs continue to drive innovation in visual perception tasks across industries.
Facial recognition technology and privacy considerations
Facial recognition technology represents one of the most powerful and controversial applications of computer vision. By analyzing facial features and patterns, AI systems can identify individuals with remarkable accuracy, opening up new possibilities in security, authentication, and personalized services. However, the widespread deployment of facial recognition also raises significant privacy concerns and ethical questions.
As facial recognition technology continues to advance, innovators are grappling with the challenge of balancing its benefits with the need to protect individual privacy. This has spurred the development of privacy-preserving AI techniques, such as federated learning and differential privacy, which aim to maintain the utility of facial recognition while safeguarding personal information.
Autonomous vehicle perception using AI vision systems
The development of autonomous vehicles represents one of the most ambitious applications of computer vision and AI. Vision systems in self-driving cars must interpret complex road environments in real-time, recognizing objects, predicting movements, and making split-second decisions. This challenge has driven significant innovations in sensor fusion, 3D scene understanding, and predictive modeling.
AI-powered perception systems in autonomous vehicles are not only advancing transportation technology but also pushing the boundaries of what's possible in real-time decision-making and environmental understanding. These innovations have broader implications for fields such as robotics, smart cities, and augmented reality, where AI-enhanced perception can improve safety, efficiency, and user experiences.
AI ethics and responsible innovation frameworks
As AI continues to permeate various aspects of society, the need for ethical considerations and responsible innovation frameworks has become paramount. Addressing the ethical implications of AI is not just a moral imperative but also a crucial factor in ensuring the long-term sustainability and public acceptance of AI technologies.
Fairness, accountability and transparency in AI algorithms
The concepts of fairness, accountability, and transparency (FAT) have emerged as key principles in the development of ethical AI systems. Ensuring that AI algorithms make fair and unbiased decisions, are accountable for their outputs, and operate in a transparent manner is essential for building trust in AI technologies.
Innovators are developing new techniques to audit AI systems for bias, explain the decision-making processes of complex models, and create more interpretable AI architectures. These efforts are crucial for the responsible deployment of AI in sensitive domains such as healthcare, criminal justice, and financial services, where algorithmic decisions can have significant impacts on individuals' lives.
Bias mitigation techniques in machine learning models
Addressing bias in machine learning models is a critical challenge in AI ethics. Bias can arise from various sources, including imbalanced training data, historical prejudices reflected in datasets, and flawed algorithm design. Innovators are developing sophisticated techniques to detect and mitigate bias at different stages of the machine learning pipeline.
Some approaches focus on preprocessing techniques to balance datasets, while others involve adjusting model architectures or loss functions to promote fairness. Post-processing methods are also being developed to calibrate model outputs and ensure equitable treatment across different demographic groups. These innovations are essential for creating AI systems that are not only powerful but also equitable and socially responsible.
AI governance and regulatory compliance strategies
As AI systems become more prevalent and influential, the need for effective governance frameworks and regulatory compliance strategies has grown. Innovators and policymakers are working to develop guidelines and standards that ensure AI technologies are developed and deployed in ways that align with societal values and legal requirements.
These efforts include the creation of AI ethics boards within organizations, the development of industry-wide standards for AI development and testing, and the formulation of legal frameworks to govern AI use. Innovations in this space are focusing on creating flexible and adaptive governance models that can keep pace with the rapid evolution of AI technologies while safeguarding public interests.
Quantum computing and AI: the next frontier of computational power
The convergence of quantum computing and artificial intelligence represents one of the most exciting frontiers in technological innovation. Quantum computers, leveraging the principles of quantum mechanics, have the potential to solve certain complex problems exponentially faster than classical computers. This quantum advantage could revolutionize AI by enabling the processing of vast amounts of data and the simulation of complex systems at unprecedented scales.
Researchers are exploring how quantum algorithms can enhance machine learning models, potentially leading to breakthroughs in optimization, pattern recognition, and data analysis. The development of quantum machine learning algorithms could unlock new possibilities in drug discovery, financial modeling, and climate prediction, tackling challenges that are currently intractable with classical computing resources.
While fully-fledged quantum computers are still in development, the progress in this field is driving innovation in hybrid quantum-classical systems and quantum-inspired algorithms. These advancements are paving the way for a new era of AI capabilities that could transform industries and scientific research in profound ways.
Ai-driven robotics and automation in manufacturing and logistics
The integration of AI with robotics and automation systems is revolutionizing manufacturing and logistics industries. AI-powered robots are becoming increasingly versatile, adaptable, and capable of performing complex tasks with minimal human intervention. This convergence is driving innovations in smart factories, warehouse automation, and supply chain optimization.
In manufacturing, AI is enabling the development of more flexible and efficient production lines. Collaborative robots (cobots) equipped with advanced computer vision and machine learning algorithms can work alongside humans, adapting to new tasks quickly and ensuring safer working environments. AI-driven predictive maintenance systems are also enhancing equipment reliability and reducing downtime, leading to significant cost savings and productivity improvements.
In logistics, AI is optimizing route planning, inventory management, and order fulfillment processes. Autonomous guided vehicles (AGVs) and drones are being deployed in warehouses and distribution centers, leveraging AI for navigation and object manipulation. These innovations are not only increasing operational efficiency but also addressing labor shortages and improving workplace safety.
The continuous advancements in AI-driven robotics and automation are paving the way for more resilient and adaptive supply chains, capable of responding quickly to market changes and disruptions. As these technologies continue to evolve, they promise to reshape the future of manufacturing and logistics, driving economic growth and competitiveness on a global scale.